Managerial Accounting Assignment Help
Managerial accounting is an interdisciplinary branch of accounting that bridges financial information with strategic decision-making processes. As an academic discipline, managerial accounting presents students with a challenging subject matter which demands proficiency in cost analysis, budgeting and financial planning concepts.
An efficient managerial accounting assignment help service can clarify complex concepts and ensure assignments adhere to academic standards, as well as provide advice on how to approach case study questions and problems.
Cost Analysis
Managerial accounting requires extensive study and insight, so having professional tutors to guide student assignments and homework completion is crucial. Our team of professionals offers online managerial accounting assistance for high school, college and graduate level students.
Cost analysis is the practice of closely inspecting costs to identify opportunities for improvement. It includes reviewing both fixed expenses like rent and salaries as well as variable costs like raw materials. Through cost analysis, Absorption and Variable Costing businesses can increase product profitability by cutting production costs without compromising quality.
Business costing can help businesses make smart decisions regarding resource allocation, pricing strategies and cost management. By understanding the behavior of costs across activity levels, managers can accurately predict future expenses and performance evaluations. Marginal costing analyzes incremental unit production costs which allow companies to determine breakeven points for different product sales mixes while also identifying more profitable ways of selling goods and services.
Budgeting
Managerial accounting is an indispensable subject that aims to provide financial insights and analysis that support decision-making within an organization. As it is such an integral component of business success, this field demands significant student attention enrolled in this course are often assigned projects which demonstrate their comprehension of managerial accounting concepts.
However, it can be challenging to complete these assignments on your own; that is where professional experts come in handy. They can assist with completing your assignments with minimal effort and cost incurred as well as provide tips and guidance that will increase your managerial accounting knowledge.
These professionals provide services on time and within your predetermined deadline. Additionally, Activity-Based Costing they can help explain complex accounting topics in easy terms while offering you an in-depth report that will enhance your performance and help you earn higher grades in managerial accounting.
Financial Statement Analysis
Financial statement analysis is an integral component of managerial accounting. It involves studying a company’s financial statements to uncover trends and identify issues affecting performance, as well as helping managers make more informed decisions by interpreting this information. There are various techniques for performing this analysis; Horizontal comparison includes comparing items across categories of statements; Vertical analysis involves comparing specific line items; Ratio analysis utilizes several metrics at once in order to reveal broad trends.
Financial statement analysis is a complex task requiring expert knowledge of financial statement analysis. Managerial accounting assignment help services offer students the specialized support needed to master this subject matter, Business Metrics Development including concept clarity, problem-solving assistance and writing academically standard assignments. They may even assist students in finding relevant sources and data for their assignments.
Financial Planning
Managerial accounting is an increasingly significant area of accounting practice that addresses specific internal needs in businesses or organizations. It specializes in providing financial data and analysis that allows managers to make more informed decisions within their organizations, Capital Budgeting often through research and complex calculations. Managerial accounting’s main aim is to maximize company value and profitability.
An effective and cost-efficient way to improve grades and complete assignments quickly is enlisting professional managerial accounting assignment help from a trustworthy online service. Their field experts can assist in understanding complex concepts, solving issues and writing quality papers on topics like costing, budgeting, variance analysis and financial statement analysis – plus they’ll work closely with you so that the paper meets instructor specifications!
Pay Someone To Do My Managerial Accounting Assignment
Managerial accounting assignment help services can offer assistance with various tasks related to this field, Cost such as research, essay writing and deadline management. Furthermore, these experts may offer insight into managerial accounting concepts and principles.
Understanding the fundamentals of managerial accounting is essential for those pursuing careers in business management or accounting, including aspects like product costing and performance measurement.
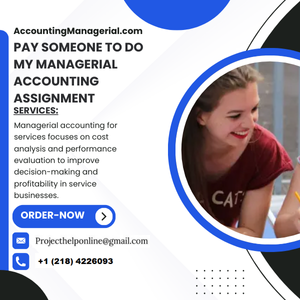
Thesis Statement
Managerial accounting is an increasingly significant field within accounting that plays an essential role in business and finance environments. To thrive at it successfully, managerial accounting students require significant understanding and application; Cost Accounting those pursuing degrees should seek professional help for assignment help in managerial accounting in order to increase their chances of scoring higher grades.
Regular accounting primarily deals with creating statements of accounts and record-keeping; managerial accounting differs substantially by providing guidance for taking expenditure or investment decisions as well as helping managers to understand potential opportunities and constraints from a financial viewpoint.
Managerial accountants play an essential role in any successful business venture, from planning and budgeting, providing cost information for decision making, identifying variance analysis and performance measurement to variance management and performance monitoring. Anyone needing help with their managerial accounting assignments should seek it from reliable online sources that provide quality assistance.
Introduction
Managerial accounting is an invaluable discipline that provides financial insights and analysis that enable managers to make more informed decisions. But its complex concepts and applications make it a difficult subject for students to grasp; those enrolled in managerial accounting courses often receive assignments to demonstrate their grasp of this complex field.
Assignments that require interpretation and calculations may be complex and time-consuming for students to complete, often necessitating extensive research with multiple citations requiring management by them.
As such, some assignments require software, further complicating their completion on time and achieving high grades. To prevent this scenario from unfolding, Cost-Volume-Profit Analysis students should seek managerial accounting assignment help from a reliable service. Such services offer many advantages to students including:
Body
Managerial accounting encompasses the examination, Data Analysis presentation, recognition and recording of financial data used for decision making, planning and overall control within an organization. Additionally, managerial accounts and reports that provide timely statistical information enable managers to make critical decisions within their organizations.
Financial accounting primarily deals with external reporting for investors, creditors, and regulators while managerial accounting concentrates more on internal control and decision-making. Students of managerial accounting learn about variable, fixed, direct, indirect costs as well as how they can be used to make better decisions; additionally it covers topics like budgeting, variance analysis and capital budgeting.
Students often struggle with managerial accounting because it requires an in-depth knowledge of accounting theory and concepts than other subjects. Writing assignments on this topic often requires extensive research time, which is why so many turn to us for our online managerial accounting homework help solutions at reasonable rates. Our experts offer tailored solutions at unbeatably fair rates.
Conclusion
Contrasting financial accounting’s focus on external reporting for investors and creditors, managerial accounting aims at aiding internal planning, control, and decision-making processes. This encompasses concepts like cost behavior (understanding how different costs shift in response to production levels or activity), cost-volume-profit analysis (examining relationships among various factors), budgeting, variance analysis.
Assignments in this subject often involve solving problems and interpreting data, which can be challenging for students. Furthermore, assignments often necessitate conducting extensive research and analysis – something which takes up significant amounts of time – as well as using specific accounting software which adds a layer of complexity.
There are various online services offering managerial accounting assignment help to students, which provide research, writing, reviewing, Forecasting editing and deadline management assistance as well as individualized tutoring sessions that help students grasp complex concepts better and increase understanding.
Benefits of Our Online Exam Help
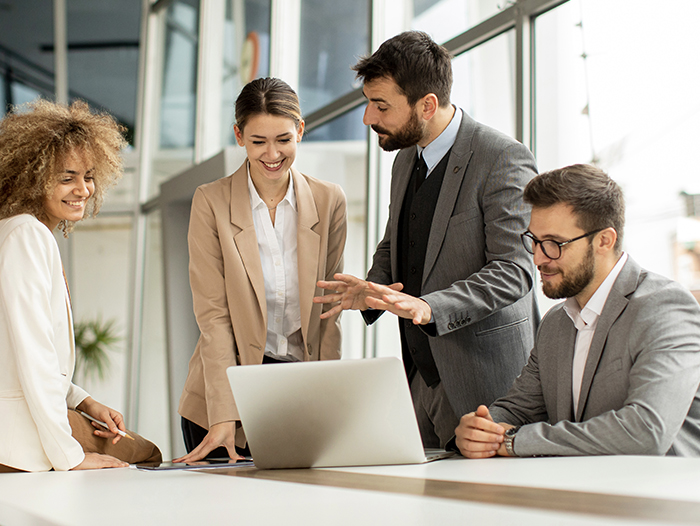
Expert Assistance
Expert assistance offers specialized knowledge and skills to help you navigate complex tasks, ensuring high-quality results. With professional guidance, you can achieve optimal solutions efficiently and effectively.
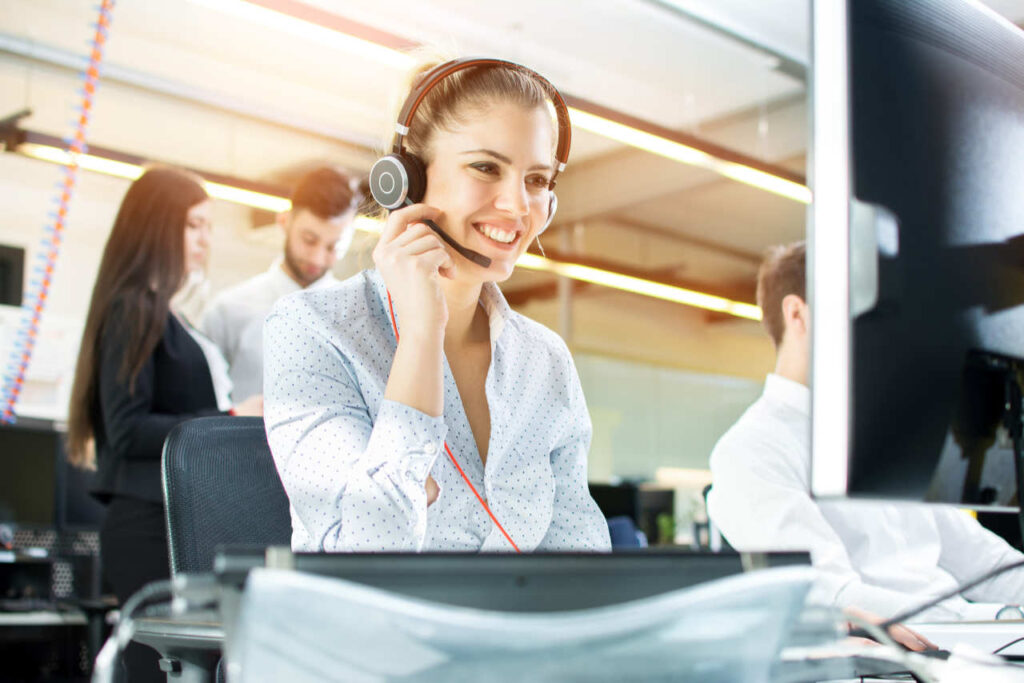
24/7 Support
24/7 support ensures that help is always available, no matter the time of day or night. Whether it’s troubleshooting or guidance, expert assistance is just a click away, around the clock.
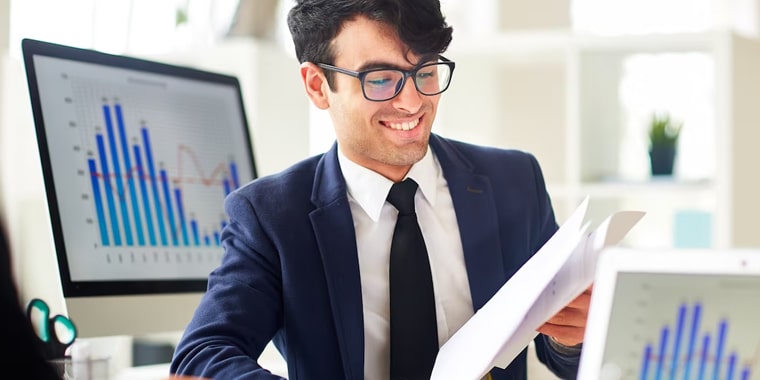
Time and Cost-Efficient
Time and cost-efficient services streamline tasks, saving you valuable time while reducing expenses. By leveraging expert solutions, you achieve quicker results without compromising quality or budget.
Hire Someone To Take My Managerial Accounting Assignment
Managerial accounting can be an intimidating topic for students, Improving Profits since its complexity requires considerable time to learn and comprehend. Professionals can assist you with your managerial accounting homework by offering tips and guidance to improve assignments.
These professionals will assist with key concepts like product costing, performance evaluation and budgeting that will enable you to secure high marks on assignments.
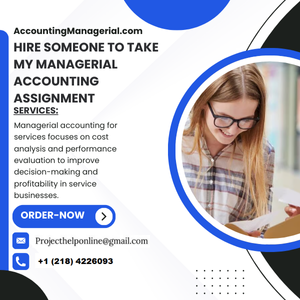
Cost Behaviour
Management of any business must understand how costs respond to changes in activity levels within their organization. This knowledge is critical for budgeting, cost-volume-profit analysis and controlling costs; cost behavior analysis involves the study of mathematical cost functions.
An audit is also crucial in creating a budget and forecasting future conditions, Inventory Methods while helping management increase profit margins by controlling costs.
Some expenses can be divided into fixed and variable expenses, with some including both elements. For instance, bike shops pay their sales staff both a monthly salary plus commission on every sale of bikes sold; these costs should be classified as fixed costs with variable costs being commissions; their management must understand how these various costs behave to improve short-term decision-making which in turn leads to greater profits and business expansion; they will also need this knowledge when planning for unexpected shifts in activity levels.
Budgeting
Managerial accounting involves gathering and disseminating information that will assist a company in increasing its internal efficiency. It seeks to enhance internal financial metrics like sales revenue and cost controls while simultaneously helping companies craft budgets and manage cash flow more effectively. Managerial accounting also aids managers in analyzing business opportunities that will increase company profits.
Managerial accountants provide different information for different departments. For instance, production department managers might prefer seeing monthly production data while HR would likely appreciate an overview of employee salaries. Since managerial accounting serves internal users best, its presentation can be tailored specifically to suit every department’s requirements.
Managers require access to high-quality financial data and insights. This can be achieved by making sure information is accessible, visualised in an easily understandable format and actively monitored against budget outcomes for any deviations from plan. Furthermore, Managerial Accounting companies should monitor budget outcomes closely so as to take any necessary actions as soon as potential deviations from plans arise.
Performance Measurement
Performance measurement systems are ongoing organizational processes that utilize critical data in order to track it over time and provide a powerful feedback loop on how well departments within a company are functioning as well as to highlight areas that management considers essential for growth. They may fall under staff responsibility, useful reference serving as an invaluable feedback loop about how well employees and departments within an organization are functioning overall and giving priority attention to those areas which management deems critical for its growth.
Evaluation criteria vary based on both industry and department. A manufacturer might look at metrics such as production downtime, throughput rates and on-time delivery rates; whereas field services divisions might track aspects like first time fix rates and service level agreement compliance rates.
Companies using metrics can use them to compare actual results with budgeted targets, and monitor any positive or negative deviations from budgeted amounts, Ratio Analysis known as variance analysis. It lays the groundwork for decision-making by bringing big-picture objectives into focus for managers and employees – ultimately increasing goal congruence and encouraging high performance.
Decision-Making
Financial accounting generally produces reports targeted to external stakeholders; managerial accounting provides documents to assist managers in understanding and analyzing internal company data to make more informed decisions and develop plans; this may involve setting goals, controlling costs or planning how best to achieve those goals.
Managerial accounting skills are invaluable for anyone pursuing a career in business. A manager might decide to expand sales territories and thus increase profits; Absorption and Variable Costing making such a decision requires having an excellent understanding of both the market and how best to communicate with members of your finance team.
Managers use managerial accounting knowledge to make informed decisions regarding long-term assets and major capital investments – often known as capital budgeting – while their knowledge also allows them to assess how events may have an effect on financial health of an organization. But ultimately, decision making remains at the core of managerial accounting.